Table Of Content
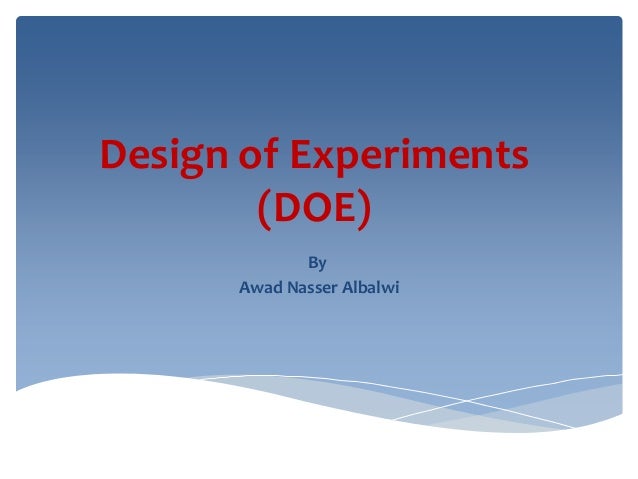
Design of Experiments terminology is more clearly understood when applied to a practical example. Suppose a project team used DOE to optimize the process for baking a cake. Six Sigma Design of Experiments is a systematic process that breaks down the variables of production and analyzes each one. This process has its own set of terms that we must understand to become conversant with how the technique works. Learn how to define the mean in simple terms and master the basics of this essential statistical concept for data analysis and interpretation.
Product Design
Therefore, a complete experimental plan consists of the combination of factors used to evaluate their effects on the response. An alternative scenario might occur if patients were randomly assigned treatments as they came in the door. At the end of the study, they might realize that drug A had only been given to the male subjects and drug B was only given to the female subjects.
main types of Design of Experiments (DOE) designs
Most DOE software, especially our own, is also there to help guide you. They can also help you identify when technical restrictions apply and avoid making the wrong choice. This translates into a small amount of additional experimental work and can be a useful pattern when iterating from stage to stage of your DOE campaign. This number tells you what “order”—or in non “DOE speak”, the size—of interactions you can distinguish within a particular effect.
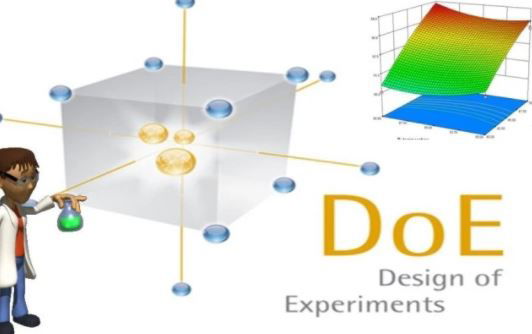
You can determine optimal settings for your variables
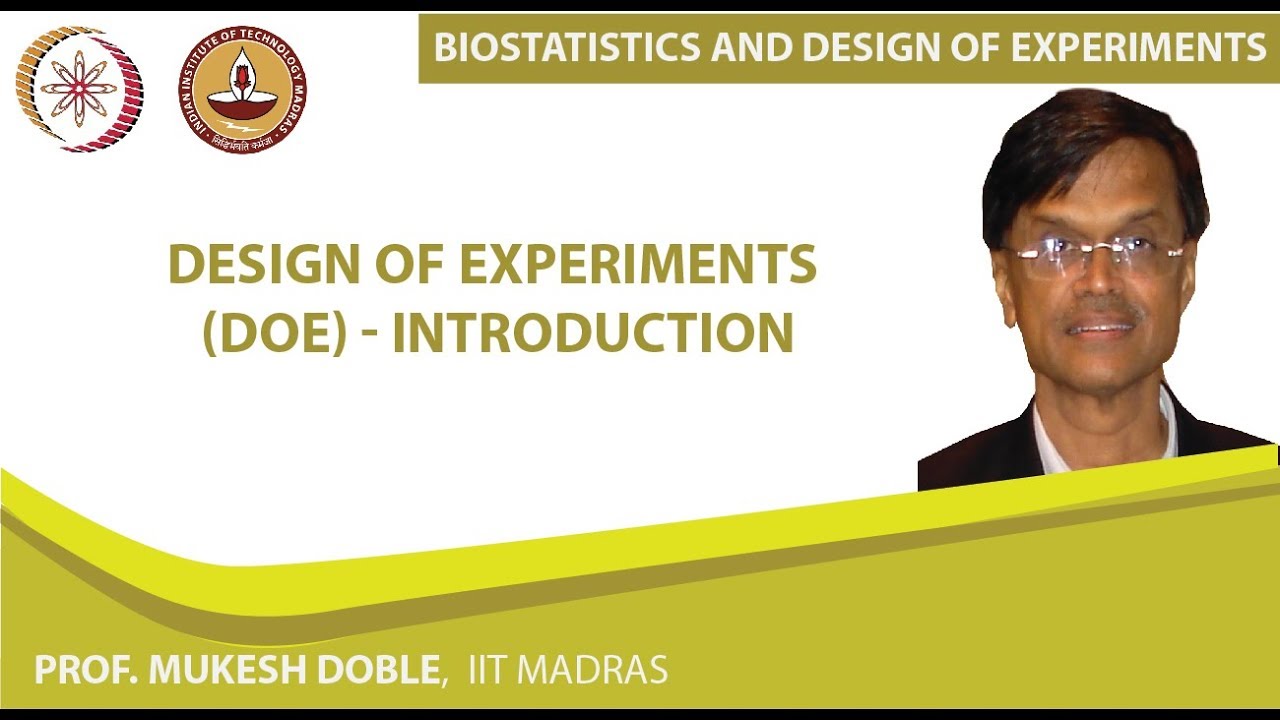
In reality, there are nuances to the different types of RSM designs. The main effects of a DOE are the individual factors that have a statistically significant effect on your output. In the common two-level DOE, an effect is measured by subtracting the response value for running at the high level from the response value for running at the low level. Unless you’ve done some prior screening of your potential factors, you might want to start your DOE with a screening or fractional factorial design. This will provide information as to potentially significant factors without consuming your whole budget.
It was at this time that Box and Wilson (1951) wrote the key paper in response surface designs thinking of the output as a response function and trying to find the optimum conditions for this function. He worked in the chemical industry in England in his early career and then came to America and worked at the University of Wisconsin for most of his career. It is used in most experiments because it is simple, versatile and can be used for many factors.
Design of Experiment Strategy for an Electrospray Ionization Optimization in Supercritical Fluid Chromatography–Mass ... - LCGC Chromatography Online
Design of Experiment Strategy for an Electrospray Ionization Optimization in Supercritical Fluid Chromatography–Mass ....
Posted: Tue, 01 Oct 2019 07:00:00 GMT [source]
By KnowledgeHut .
This allows us to optimize the performance and robustness of our processes or assays. DOE requires fewer resources for the amount of information obtained, saving on time and materials. By measuring multiple factors at once, you are reducing the number of biological and technical replicates required for a statistically accurate measurement compared to measuring those factors individually.
Bioreactor systems enhanced with software for Design of Experiments - Scientist Live
Bioreactor systems enhanced with software for Design of Experiments.
Posted: Thu, 26 Feb 2015 08:00:00 GMT [source]
Once the problem is defined, you can begin planning for the experiment. This entails determining the factors to be varied, the expected responses, experimental procedures, and equipment to be used. If the number of factors is large, you may need to perform screening experiments to choose the most crucial ones. There are different design types that you can use to achieve this, such as fractional factorial design, Plackett-Burman design, and definitive screening design.
Process Improvement
Throughout this exploration of the Design of Experiments (DoE), we’ve unveiled the methodology’s profound capability to refine research methods, enhancing precision in data analysis and discovering inherent truths. From ensuring unbiased data through randomization and enhancing experimental reliability via replication to the meticulous design showcased by blocking, DoE embodies a holistic approach to scientific inquiry. It rests on a philosophical foundation that values truth in measurement, goodness in methodology, and beauty in data visualization, all while upholding the highest ethical standards. This journey through DoE’s essential components, varied experimental designs, and innovative software tools, punctuated by a case study, illustrates its transformative impact across fields.
Machine Learning (ML), whereby computational algorithms interpret complex data, is a methodological approach to solving optimization problems when there is a lot of data available. DOE can help ML approaches become more effective by finding the optimal algorithmic parameter settings, while ML can support DOE by better detecting the effects of factors and their interactions. Biological experimentation can be expensive, but through the use of DOE, coupled with ML, it may be possible to build Machine Learning capabilities using smaller (and less costly) data sets. This is especially useful as experiments scale up and the amount of data generated is difficult to collect and process manually.
DOE can be difficult to plan and analyze; however experimental execution of a DOE can be particularly challenging, especially for those less comfortable with automation. Whatever software package you use, there is plenty of support and information to help you design and analyze your experiment. In contrast to OFAT experimentation, the systematic structure of experimental conditions in DOE allows researchers to vary and test multiple factors in one go.
You can either use full factorial designs with all possible factor combinations, or fractional factorial designs using smaller subsets of the combinations. A design of experiments (DOE) is a set of statistical tools for planning, executing, analyzing, and interpreting experimental tests to determine the impact of your process factors on the outcomes of your process. With three variables, machine speed, fill speed, and carbonation level, how many different unique combinations would you have to test to explore all the possibilities? Which combination of machine speed, fill speed, and carbonation level will give you the most consistent fill? The experimentation using all possible factor combinations is called a full factorial design. Some efficient designs for estimating several main effects were found independently and in near succession by Raj Chandra Bose and K.
Design of Experiments (DOE) is a branch of applied statistics focused on using the scientific method for planning, conducting, analyzing and interpreting data from controlled tests or experiments. DOE is a mathematical methodology used to effectively plan and conduct scientific studies that change input variables (X) together to reveal their effect on a given response or the output variable (Y). In plain, non-statistical language, the DOE allows you to evaluate multiple variables or inputs to a process or design, their interactions with each other and their impact on the output. In addition, if performed and analyzed properly you should be able to determine which variables have the most and least impact on the output.
Conducting experimental design allows you to look at different alternatives. It helps in making an informed decision on what to use or what to change. This methodology can also be used to discover the best combination of alternatives in the experiment. The key concept behind this methodology is that there is a relationship between the factors affecting the response. ISixSigma defined it as determining the “cause and effect relationships” of factors.
You could include intrinsic noise as a response to be minimized alongside your measures of the output’s quality and quantity. We can often find aggregate measures (such as in the yield example above) that avoid the need to dissect how our system does what we want in too much detail. But it’s usually necessary to include more than one response in our optimization. In technical terms, this makes our problem one of multi-objective optimization. We already touched on one version of this above, where we tried to achieve a high yield with a low level of impurities.
In DoE, experiments are being used to find an unknown outcome or effect, to test a theory, or to demonstrate an already known effect. They are done by scientists and engineers, among others, in order to understand which inputs have a major impact on output and what input levels should be targeted to reach a desired outcome (output). Simply put, DoE is a way to collect information during the experiment and then determine what factors or which processes could lead to the desired result. When factors or levels increase, full factorial designs can become infeasible—even in sophisticated, high-capacity facilities.
Though you’ll find that DOE software will often prevent you from making certain choices that your design does not permit. Instead of trying to fit your DOE campaign into a single experiment, think of the DOE process as a collection of sub-experiments. And for each sub-experiment, you might use a different design—as every type of DOE design at each step is intimately linked to a phase in the DOE campaign. They can move you rapidly from your initial “thought experiment” to optimized conditions and robust data. Notice that none of them has trials conducted at a low temperature and time AND near optimum conditions. Test different settings of two factors and see what the resulting yield is.
No comments:
Post a Comment